Understanding Bounding Boxes in Data Annotation for AI
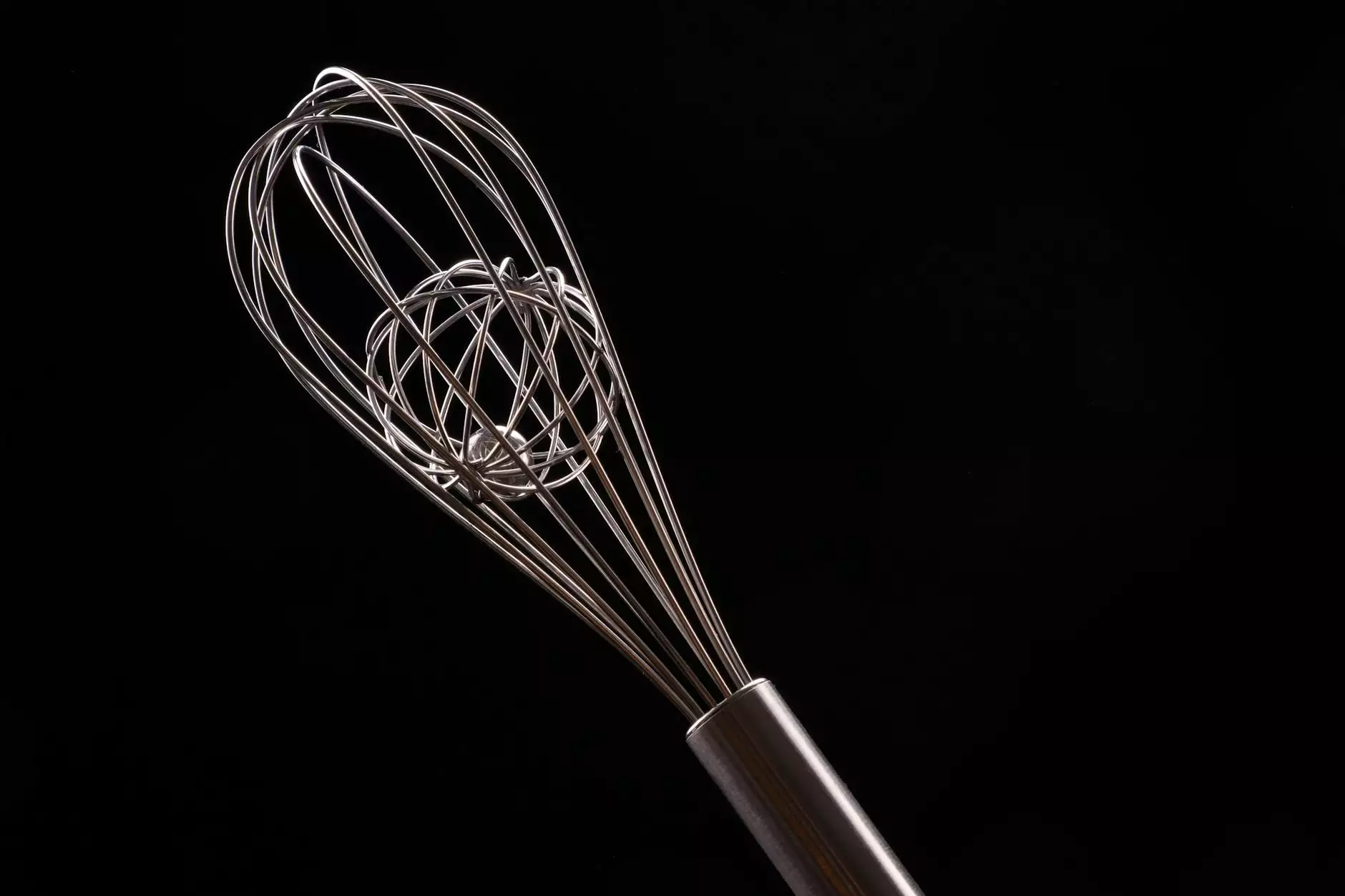
In the realm of artificial intelligence and machine learning, data annotation plays a crucial role in training algorithms to recognize patterns and make decisions. Among the various methods of data annotation, the concept of bounding boxes has emerged as a fundamental technique, especially in the fields of computer vision and image processing. Understanding how these rectangular frameworks enhance AI capabilities can illuminate their importance in the vast landscape of data-driven projects.
What Are Bounding Boxes?
Bounding boxes are rectangular boxes used to delineate an object within an image or video frame. This simplistic yet powerful tool serves as a fundamental building block for numerous applications in computer vision. Essentially, a bounding box represents the location of an object by providing a minimal enclosing rectangle that aids in identifying and classifying that object, often referred to in the context of object detection.
The Anatomy of Bounding Boxes
A bounding box is characterized by two primary components:
- Coordinates: The bounding box is defined by the coordinates of its upper-left and lower-right corners (x-min, y-min, x-max, y-max). This information helps locate the bound object in the image space.
- Label: Bounding boxes are often accompanied by labels that provide context for the objects they contain, such as "car," "person," or "dog."
The Importance of Bounding Boxes in Data Annotation
The use of bounding boxes in data annotation serves several critical purposes:
1. Accurate Object Localization
Bounding boxes enable precise localization of objects within images, allowing algorithms to not only identify objects but also understand their positional context. By training on annotated images that feature bounding boxes, AI models become adept at recognizing the spatial arrangement of various entities.
2. Enhanced Learning for Deep Learning Models
The incorporation of bounding boxes in training datasets is particularly beneficial for deep learning models, such as convolutional neural networks (CNNs). These models excel at extracting features from images, and bounding boxes provide a structured way to learn from the visual data while minimizing noise, ultimately enhancing the model's performance.
3. Support for Multiple Object Detection
Bounding boxes facilitate the detection and differentiation of multiple objects in a single frame. This capability is essential for applications ranging from traffic monitoring to surveillance and autonomous vehicles, where understanding the dynamics between various objects is crucial.
The Process of Data Annotation Using Bounding Boxes
Annotating data with bounding boxes involves several key steps:
Step 1: Image Selection
The first step in the data annotation process is selecting a diverse set of images that represent the relevant categories. Diversity ensures that the AI system can generalize well across various scenarios.
Step 2: Manual Annotation or Automated Tools
Once the images are selected, the annotation team has the option of manually drawing bounding boxes around the objects or utilizing advanced annotation tools, such as those offered by Keylabs.ai. These tools often feature high accuracy and user-friendly interfaces that streamline the process.
Step 3: Quality Assurance
The annotated data must undergo rigorous quality assurance checks to ensure that the bounding boxes accurately encapsulate the objects and the labels are correctly assigned. This step is fundamental to building reliable models.
Utilizing Keylabs.ai for Efficient Data Annotation
At Keylabs.ai, we understand the complexities surrounding data annotation. Our Data Annotation Tool and Data Annotation Platform are designed to simplify and enhance the bounding box annotation process. Here’s how:
- User-Friendly Interface: Our platform offers an intuitive interface that allows annotators to create bounding boxes swiftly and accurately.
- Collaboration Features: Multiple team members can work on the same project simultaneously, ensuring faster project turnaround times.
- Quality Control Mechanisms: Built-in quality checks allow for real-time review of annotations, reducing error rates and improving overall dataset reliability.
- Scalability: No matter the size of the project, our platform can scale seamlessly to accommodate large datasets for businesses and organizations.
Applications of Bounding Boxes Across Industries
Bounding boxes have found applications in various sectors, each leveraging their unique capabilities:
1. Autonomous Vehicles
In the automotive industry, bounding boxes are integral to self-driving car technology. They help the vehicle’s AI system detect and navigate surrounding vehicles, pedestrians, and obstacles, ensuring safer travel.
2. Retail and Inventory Management
Retailers utilize bounding boxes in computer vision systems to monitor stock levels and analyze consumer behavior, allowing for efficient inventory management and improved customer experiences.
3. Healthcare
In healthcare, bounding boxes assist in analyzing medical images to identify abnormalities or anomalies—such as tumors in CT scans—by training AI algorithms on annotated datasets.
Challenges and Considerations in Bounding Box Annotation
While bounding boxes are incredibly useful, their implementation does come with challenges:
1. Complexity of Object Shapes
Many objects have irregular shapes that a rectangular box may not fully encapsulate. In such cases, more advanced annotation techniques, like polygonal segmentation, may be required to accurately represent the object.
2. Subjectivity in Labeling
There can be inherent subjectivity in how bounding boxes are drawn and labeled, which can lead to inconsistencies across the dataset. Rigorous annotation guidelines and quality checks are essential to mitigate this issue.
3. Time and Resource Intensive
Manual annotation is often time-consuming and resource-intensive. Investing in automated solutions or platforms, like Keylabs.ai, can significantly enhance efficiency.
The Future of Bounding Boxes and AI
The future of bounding boxes in AI is promising as advancements in technology continue to evolve. Here are some anticipated trends:
1. Integration of AI in Annotation
AI-driven tools are expected to automate the annotation process further, allowing for rapid generation of bounding boxes with high accuracy, significantly reducing the need for manual input.
2. Enhanced Algorithms
The development of more sophisticated algorithms will likely enable better object detection, classification, and localization even in complex environments, making bounding boxes even more effective.
3. Expanding Application Areas
As AI continues to permeate various industries, the utilization of bounding boxes will expand, potentially finding new applications in areas such as augmented reality (AR) and virtual reality (VR), where understanding the geometric relationships of objects is crucial.
Conclusion: The Integral Role of Bounding Boxes in AI Development
Bounding boxes are a pivotal element of data annotation in the field of artificial intelligence. Their ability to accurately outline and classify objects enhances the efficacy of machine learning models, which is essential for numerous real-world applications. By leveraging advanced data annotation tools, such as those provided by Keylabs.ai, businesses can significantly improve the quality of their datasets, leading to more robust AI solutions.
As we look toward the future, the evolution of bounding boxes alongside burgeoning AI technologies promises exciting developments that will continue to transform industries and enhance capabilities across the board.