All About ML: Understanding Machine Learning and Its Impact on Business
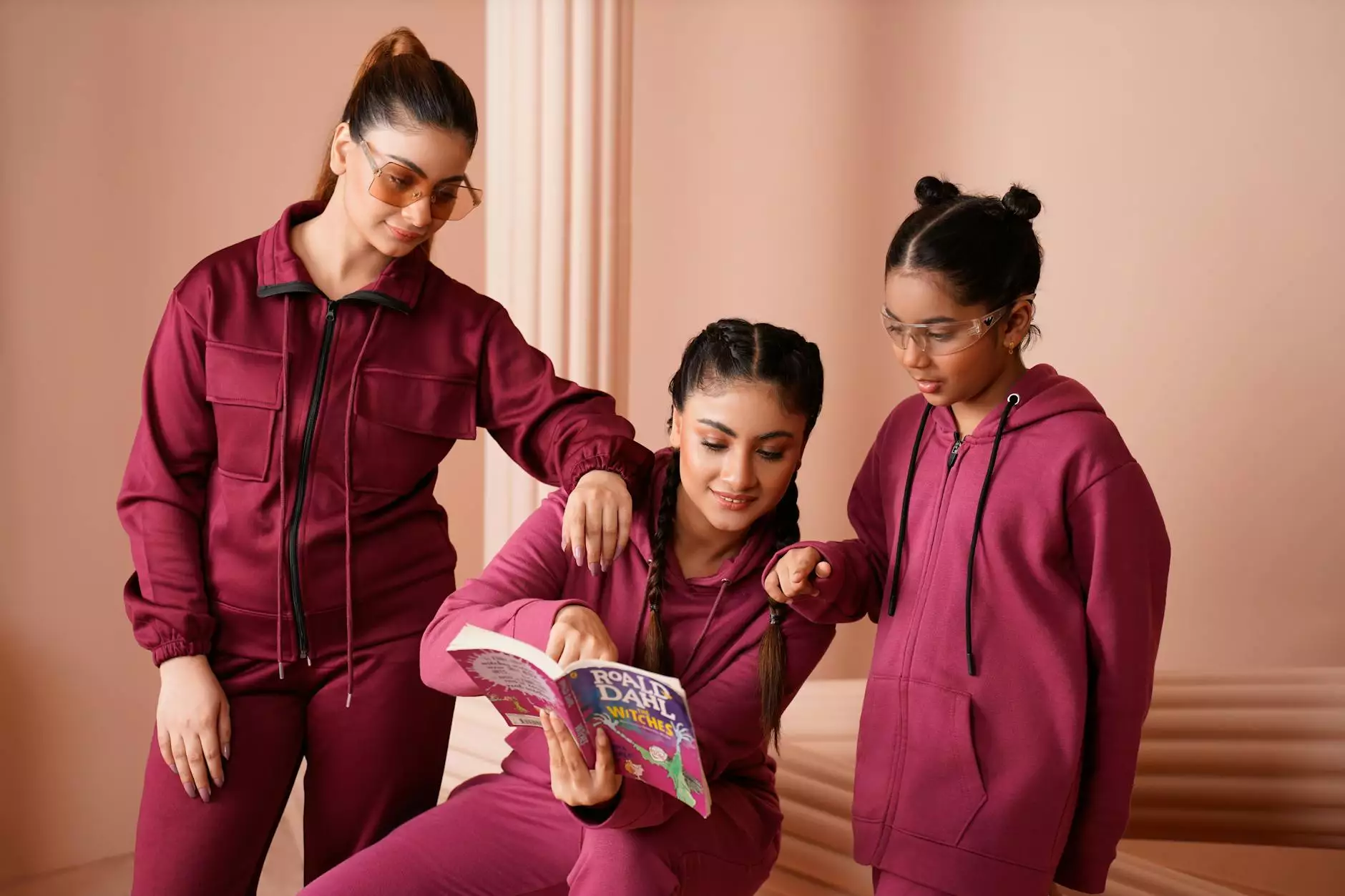
In today's digital age, machine learning (ML) has emerged as one of the most transformative technologies influencing various industries. From healthcare and finance to retail and entertainment, the applications of ML are remarkable and far-reaching. This article, all about ML, will delve deep into the complexities and benefits of machine learning, especially in the realm of business.
What is Machine Learning?
At its core, machine learning is a subset of artificial intelligence (AI) that enables systems to learn from data, identify patterns, and make decisions with minimal human intervention. Unlike traditional programming where explicit instructions are given to a system, ML algorithms use statistical techniques to provide computers with the ability to "learn" from previous computations and improve over time.
The Basics of Machine Learning
Machine learning involves several key processes that are vital for its implementation:
- Data Collection: The first step is gathering relevant data from various sources.
- Data Preparation: This involves cleaning and organizing data for analysis.
- Model Training: Here, algorithms are employed to learn from the data, creating models that can make predictions.
- Model Evaluation: Assessing the accuracy of the model based on how well it performs against unseen data.
- Deployment: Finally, the trained model is implemented into real-world applications.
The Different Types of Machine Learning
Understanding the different types of machine learning is crucial in grasping how to apply it effectively in business. The primary categories include:
1. Supervised Learning
In supervised learning, algorithms learn from labeled datasets, which means the input data is paired with the correct output. This method is particularly useful for regression and classification tasks. Businesses can use this approach to predict customer behavior or classify emails as spam or not.
2. Unsupervised Learning
Unsupervised learning involves training a model on data without labeled responses. The algorithm tries to learn the underlying structure of the data on its own, making it invaluable for tasks such as clustering and association. Businesses can leverage unsupervised learning for customer segmentation or market basket analysis.
3. Reinforcement Learning
Reinforcement learning is a type of learning where an agent interacts with its environment and learns to maximize some notion of cumulative reward. This approach is widely used in areas such as robotics, gaming, and navigation systems in transportation.
Why is Machine Learning Important for Businesses?
The importance of machine learning in business cannot be understated. Here are several compelling reasons why companies should consider integrating ML into their operations:
- Informed Decision Making: ML provides businesses with insights derived from data, enabling them to make informed decisions based on predictive analytics.
- Increased Efficiency: Automating repetitive tasks through machine learning leads to significant improvements in efficiency, allowing employees to focus on higher-level tasks.
- Enhanced Customer Experience: Through personalized recommendations and targeted marketing, businesses can improve customer satisfaction and loyalty.
- Cost Reduction: ML can help identify inefficiencies and optimize processes, leading to significant cost savings over time.
- Competitive Advantage: Companies that adopt machine learning ahead of competitors can leverage advanced technologies for better strategic positioning.
Applications of Machine Learning in Business
Businesses across various sectors are harnessing the power of machine learning to innovate and drive growth. Here are some notable applications:
1. Marketing and Sales
In the marketing domain, ML algorithms analyze consumer behavior patterns, enabling businesses to tailor their advertising strategies effectively. Predictive analytics can forecast sales and help identify which marketing campaigns will yield optimal returns.
2. Financial Services
The financial sector uses machine learning for credit scoring, fraud detection, and risk management. By analyzing historical transaction data, ML models can identify fraudulent transactions in real-time, protecting businesses and consumers alike.
3. Healthcare
Machine learning is revolutionizing healthcare by enhancing diagnostics, personalizing treatment plans, and predicting patient outcomes. Algorithms analyze medical images, genetic data, and patient histories to assist healthcare providers in making better clinical decisions.
4. Supply Chain Management
In supply chain management, machine learning optimizes inventory levels and forecasting demand. By analyzing various factors, such as market trends and historical sales data, businesses can streamline their supply chains and reduce costs significantly.
5. Human Resources
HR departments leverage machine learning to streamline recruiting and employee management processes. Through predictive analytics, businesses can identify the best candidates for job openings or predict employee turnover rates.
Challenges and Considerations When Implementing Machine Learning
While the benefits of machine learning are substantial, businesses must navigate several challenges during implementation:
- Data Quality: The success of ML algorithms heavily relies on the quality of data. Inaccurate or incomplete data can lead to faulty predictions.
- Integration: Integrating machine learning systems with existing IT infrastructure can be complex and may require substantial investment.
- Talent Gap: There is a notable shortage of skilled professionals who can build and manage ML systems, making talent acquisition a significant barrier.
- Ethical Considerations: Utilizing ML raises ethical issues such as algorithmic bias, data privacy, and the potential for misuse of technology.
Future of Machine Learning in Business
As we look to the future, the role of machine learning in business is poised to expand even further. With advances in computing power, availability of big data, and enhancements in algorithm efficiency, we can expect to see:
- Increased Automation: More processes will become automated, allowing businesses to operate more efficiently.
- Greater Personalization: Businesses will continue to tailor their offerings to individual customer preferences.
- Real-Time Insights: ML will increasingly provide real-time data analytics, allowing for quick reactions to changing market conditions.
- AI Collaboration: Machine learning will work more closely with other AI technologies, like natural language processing and computer vision, creating multifaceted solutions.
Conclusion
In summary, machine learning holds significant potential for businesses looking to innovate and improve their operations. Understanding all about ML, from its basic concepts to its applications and challenges, empowers organizations to harness this technology effectively. By embracing machine learning, companies can not only enhance their decision-making processes but also ensure they remain competitive in an increasingly digital world. With careful consideration and strategic planning, the future of machine learning in business looks incredibly promising.